In 1950, Alan Turing intrigued scientists and science fiction fans alike by posing the question, “Can machines think?” In the 1968 film, “2001: A Space Odyssey,” HAL 9000 took viewers on a spectacular adventure through space, and today the world routinely engages in conversations with ChatGPT.
Although Artificial Intelligence (AI) has evolved over the past 70 years and has been embraced by some members of the health care community, the HexAI Research Laboratory in the Department of Health Information Management (HIM) stands alone.
According to Health Informatics (HI) Assistant Professor Ahmad P. Tafti, director of the Pitt HexAI Research Laboratory, “The HexAI Lab not only combines AI strategies with clinical data, it also improves AI-powered clinical applications by promoting AI explainability and interpretability.”
HexAI stands for HEALTH + EXPLAINABLE AI
Explainable AI uses scientific data along with machine learning to solve problems.
Using AI to Solve Health Care Problems
Tafti and his team focus on engineering, implementing, validating and deploying cutting-edge fundamental and applied AI and machine learning algorithms to solve real health care problems, mainly in musculoskeletal diseases and disorders.
The lab focuses on harnessing and analyzing medical images from the local medical community as well as publicly available imaging datasets, such as those provided by the National Institutes of Health.
With AI explainability, the lab develops scientific visualization methods to clearly find patterns and differences among various models and to help make decisions.
“Health care providers are accountable for the care they provide,” says Tafti. “Explainable AI can help ensure that AI systems are also held accountable.”
“The HexAI Lab aims to advance research in musculoskeletal disorders and total joint arthroplasty with the power of explainable AI,” he continues.
“In the current health care environment, image analysis is prone to variations in individual interpretations of the same image,” notes Dr. F. Johannes Plate, HexAI clinical associate director and director of Adult Reconstruction Research, Department of Orthopaedic Surgery, University of Pittsburgh.
“The development of AI algorithms that identify musculoskeletal conditions can improve diagnostic accuracy,” he continues.
“In the field of total joint replacement, explainable AI has the ability to diagnose stages of degenerative cartilage loss to allow clinicians to recommend an appropriate patient-specific treatment plan,” says Plate.
Health Informatics graduate student Ismaeel Siddiqui helps to curate fully annotated medical image datasets that help provide a precise and accurate reference point for AI models to learn from.
“With more accurate annotations, the AI models are better able to identify the specific regions of interest and make more accurate predictions,” says Siddiqui.
A multi-disciplinary team of experts, including data scientists, health informaticians, surgeons and other clinicians meet to review and discuss data. “We believe we are developing the gold standard in explainable AI,” says Tafti.
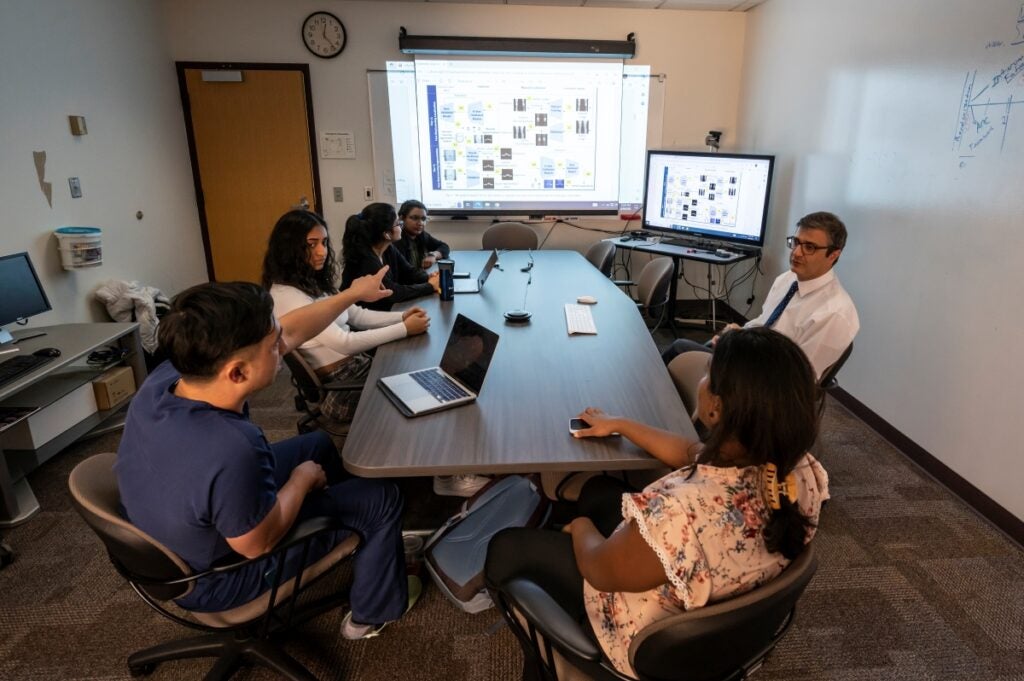
Enhancing Personalized Care
Yanshan Wang, HI assistant professor and vice chair of Research, serves as an advisory board member of the HexAI Lab. Among other things, he helps to shape research activities with meaningful deliverables and oversees the dissemination of research findings.
“When we integrate a patient’s electronic health record with other health care data sources, we can provide a more comprehensive view of that patient’s health status,” says Wang. “This integrated data can then be used to develop more sophisticated AI models that can predict health outcomes with greater accuracy and provide personalized care recommendations based on individual patient characteristics.”
Physicians can spend less time acquiring all the necessary clinical information and more time discussing specific treatment options with the patient.
“It is rewarding to know we are developing AI algorithms for personalized treatment in musculoskeletal settings that patients and providers can clearly understand so that we can optimize health outcomes,” adds HI graduate student Deanelle Thompson.
When it comes to patient care, accountability and explainability are vital. As lab assistant and HI student Christina Letter points out, “Our work can help ensure that AI systems are accountable for their decisions and that potential decision-making issues can be traced back to their source.”
“Building accountable and explainable models also builds trust with the providers who use them by allowing them to clearly understand how AI systems arrive at their decisions,” says Letter.
Award Winning
In its first year of operation, the HexAI Lab received an award from the University of Pittsburgh Clinical and Translational Science Institute (CTSI), part of the National Institutes of Health (NIH) nationwide network that provides the support necessary to bridge the gap between research and effective clinical and public health practice and policy. It was also tapped for the prestigious Oracle for Research Award.
“We believe explainable AI is the model for the future,” Tafti continues. “We are driven to deliver clear reliable data, a proven model of research, and a way to explore and solve problems that are meaningful and make the most positive impact on people’s lives.”
HexAI Podcast
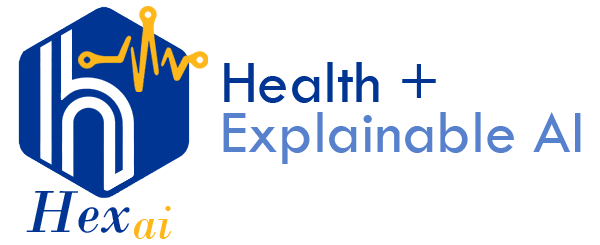
The HexAI podcast series offers a professional discussion forum for health care data scientists, students and AI practitioners to present, share and discuss the latest research findings, innovations, challenges and opportunities in digital health sciences and AI-powered health care